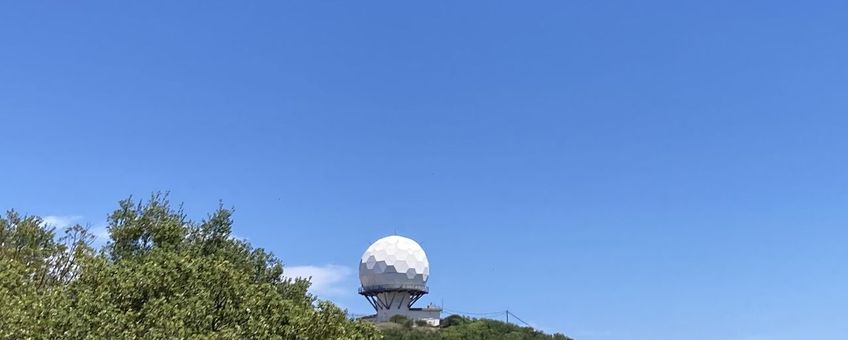
New model offers opportunity to protect migrating birds
Institute for Biodiversity and Ecosystem Dynamics (IBED)The research is a collaboration between ecologists from the Institute for Biodiversity and Ecosystem Dynamics (IBED) and computer scientists from the AI4ScienceLab. First author Fiona Lippert is associated with both institutes for her PhD research in Artificial Intelligence. "During migration birds travel through a variety of landscapes, many of which are dominated and shaped by humans," says Lippert. "This inevitably leads to conflicts – think of collisions with aircraft, buildings, power lines, or wind turbines, and the transmission of infectious diseases to humans or livestock."
Trade-off between impact and cost
To effectively prevent these conflicts, stakeholders need to know several days in advance when and where birds will fly. Lippert: "For example, wind parks can be temporarily shut down during peak migration events to reduce the number of casualties, or the illumination of buildings can be switched off at night to avoid disorientation of nocturnally migrating birds." But all these measures come at a certain cost, like the loss of wind energy during a shutdown. That is why it is vital that such a shutdown lasts as short as possible." Therefore, reliable short-term predictive models are crucial to find an optimal trade-off between maximal ecological impact and minimal financial costs."
Combining data from weather stations
Lippert and her colleagues used data from weather radars, which measure precipitation but can also record groups of birds. The researchers explored how the sparsely available data points from weather radars could be combined to make accurate predictions – both in space and time – of bird migration. They based their approach on a mathematical model that describes how the number of birds around each weather radar station changes over time as birds take off, move between neighbouring radars, and land again to rest and forage.
Processes and patterns
Lippert: "The model learned about the specific relationships between, for example, weather, landscape elements, past conditions and the different phases of migration." This allows them to replace some unknown components of the mathematical model with the outputs of neural networks: self-learning computer models. "These are trained to minimize the mismatch between bird density predictions and weather radar estimates." That resulted in FluxRGNN, a hybrid model that combines the strengths of process-based modelling and machine learning, such as understanding which processes contributed to the prediction on the one hand and finding patterns in the data on the other.
More information
- The scientific article Learning to predict spatiotemporal movement dynamics from weather radar networks is published in Methods in Ecology and can be accessed online via the link
Text: IBED
Photo: Fiona Lippert (leadfpoto: weather radar station in Corfu, Greece)